Types of Research Bias: Definition & Examples
Research bias is a systematic error that can happen at any stage of scientific investigation, from study design to data interpretation. These errors can greatly affect the accuracy and reliability of research findings, potentially leading to flawed conclusions that spread throughout the academic community. Research bias occurs when:
- Researchers unintentionally introduce systematic errors in their methodology
- Study designs favor certain outcomes over others
- Data collection or analysis methods create unintended distortions
- Personal preferences or expectations influence research decisions.
The presence of bias in academic research can undermine the basic principles of scientific inquiry. A biased study might seem methodologically sound but produce results that don't accurately reflect reality. This distortion can impact:
- The validity of research findings
- The applicability of results to larger populations
- The trustworthiness of scientific literature
- Evidence-based decision making in various fields.
Understanding different types of research bias is crucial for researchers, academics, and practitioners. By identifying potential sources of bias, you can design stronger studies, implement effective control measures, and produce more dependable research outcomes. This knowledge helps uphold the integrity of scientific research and ensures that study findings make meaningful contributions to their respective fields.
Design Bias
Design bias arises from flaws in the structure and organization of the research methodology. This systematic error can undermine the validity of the entire study before data collection even starts. Common signs of design bias include:
- Inadequate control groups that don't match experimental groups
- Poor randomization procedures
- Inappropriate measurement tools
- Insufficient sample sizes
- Lack of proper blinding techniques
Design bias can have a significant impact on study outcomes by introducing systematic errors that distort results. For example, a poorly designed questionnaire might lead participants toward specific answers, while improper selection of control groups can either hide or exaggerate treatment effects.
Key strategies to reduce design bias:
- Conduct thorough literature reviews to identify potential design pitfalls
- Use validated measurement instruments
- Implement proper randomization techniques
- Calculate appropriate sample sizes through power analysis
- Create detailed protocols for data collection
- Establish clear inclusion and exclusion criteria
- Seek peer review of research design before implementation
Research teams must identify potential design biases during the planning phase. Early detection allows for adjustments in methodology that can strengthen the study's internal validity and ensure more reliable results.
Sample Bias
Sample bias occurs when research participants don't accurately represent the target population, leading to skewed results and unreliable conclusions. This type of bias can significantly impact the validity of research findings and limit their applicability to broader populations. Common causes of sample bias include:
- Convenience sampling (selecting easily accessible participants)
- Voluntary response bias (only motivated individuals participate)
- Insufficient sample size
- Under-representation of specific demographic groups
- Non-random selection methods.
Sample bias can distort effect sizes and limit external validity, making it difficult to generalize findings beyond the study population. A research studying depression in college students might yield different results if it only includes participants from a single university or specific academic program.
Effective techniques to minimize sample bias:
- Stratified random sampling
- Probability sampling methods
- Diverse recruitment channels
- Clear inclusion/exclusion criteria
- Power analysis for appropriate sample size determination
- Multi-site participant recruitment
- Demographic quota sampling
Implementing these strategies helps ensure your sample accurately reflects the characteristics and diversity of your target population, strengthening the reliability and generalizability of your research findings
Selection Bias
Selection bias occurs when specific subgroups have a higher likelihood of being included in a study, creating a non-representative sample. This bias can significantly distort research findings and compromise the validity of conclusions. Common Sources of Selection Bias include:
- Self-selection: When participants choose to join a study based on personal interest
- Exclusion of certain groups: Deliberately or accidentally omitting specific populations
- Loss to follow-up: Participants dropping out before study completion
- Convenience sampling: Selecting easily accessible participants.
Real-World Example: A study examining the effectiveness of a new weight loss program recruits participants solely from a high-end fitness center. This selection method excludes individuals from different socioeconomic backgrounds, creating biased results that don't represent the general population.
Effective Mitigation Strategies:
- Random assignment to treatment groups
- Stratified sampling across different demographics
- Matched-pair designs
- Clear inclusion/exclusion criteria
- Multiple recruitment channels
- Regular monitoring of participant characteristics
Selection bias differs from sampling bias by focusing on systematic errors in choosing participants rather than the method of drawing samples from a population. Implementing proper selection procedures helps ensure research findings accurately reflect the target population.
Performance Bias
Performance bias occurs when researchers' expectations or different treatment of study groups affect research outcomes. This bias shows up through subtle changes in behavior, data collection methods, or interaction patterns with participants. The Hawthorne effect is a classic example of performance bias. When participants know they're being observed, they often change their natural behaviors:
- Workers increase productivity during workplace studies
- Students perform better on tests when monitored
- Patients adhere more strictly to medication schedules during clinical trials
Researcher bias can also occur through:
- Providing extra attention to treatment groups
- Unconsciously communicating expected outcomes
- Applying inconsistent measurement standards.
Effective strategies to minimize performance bias:
- Implement double-blind protocols where both researchers and participants are unaware of group assignments
- Standardize all interactions and procedures across study groups
- Use automated data collection methods when possible
- Train multiple observers to ensure consistency
- Document all deviations from standard protocols
Research teams can improve study validity by acknowledging potential sources of performance bias and implementing strict controls throughout the data collection process.
Reporting Bias
Reporting bias occurs when researchers selectively publish or report study findings based on the nature and direction of results. This bias manifests in several ways:
- Publication bias: Studies with positive or significant results are more likely to be published
- Time lag bias: Delayed publication of negative results
- Outcome reporting bias: Selective reporting of favorable outcomes while omitting unfavorable ones
The impact of reporting bias extends beyond individual studies. It creates a skewed representation of scientific evidence in literature, leading to:
- Overestimation of treatment effects
- Waste of research resources
- Potential harm to patients when ineffective treatments appear beneficial
- Reduced trust in scientific research
- Effective strategies to combat reporting bias include:
- Pre-registering study protocols before research begins
- Following CONSORT (Consolidated Standards of Reporting Trials) guidelines
- Implementing mandatory trial registration policies
- Using comprehensive reporting checklists
- Publishing null results in dedicated journals
- Adopting open science practices with transparent data sharing
- Research institutions and journals play a crucial role in reducing reporting bias by requiring clear documentation of methodology, analysis plans, and complete result sets.
Confounding Bias
Confounding bias occurs when an external variable influences both the independent and dependent variables in a study, creating a false relationship between them. This type of bias can significantly distort research findings and lead to incorrect conclusions about cause-and-effect relationships.Consider this example: A study finds that coffee consumption correlates with lung cancer. The researchers might conclude that coffee causes lung cancer. However, smoking habits - a confounding variable - affects both coffee consumption (many smokers drink coffee) and lung cancer risk.
Common characteristics of confounding bias:
- Creates spurious associations between variables
- Masks true causal relationships
- Appears frequently in observational studies
- Can lead to overestimation or underestimation of effects
Methods to control confounding bias:
- Randomization in experimental design
- Matching participants on potential confounding variables
- Statistical adjustment during data analysis
- Stratification of study populations
Researchers can identify potential confounding variables through directed acyclic graphs (DAGs) - visual tools that map relationships between variables. This systematic approach helps maintain the validity of causal inferences in observational research.
Detection Bias
Detection bias occurs when different methods or criteria are used to assess outcomes across study groups. This systematic error can significantly impact research validity and reliability.
Common Sources of Detection Bias:
- Inconsistent measurement protocols
- Varying assessment timing between groups
- Different diagnostic criteria for different participants
- Non-standardized data collection tools
To minimize detection bias, researchers implement standardized measurement protocols:
- Blinded Assessment: Evaluators remain unaware of participant group assignments
- Uniform Data Collection: Using identical tools and methods across all study groups
- Standardized Timing: Conducting assessments at consistent intervals
- Calibrated Instruments: Regular validation of measurement tools
A real-world example of detection bias appears in medical trials where researchers might unconsciously apply stricter diagnostic criteria to the treatment group compared to the control group. This bias can lead to false conclusions about treatment effectiveness, as discussed in detail in this article.
Prevention Strategies:
- Automated data collection systems
- Pre-defined assessment criteria
- Regular evaluator training
- Quality control checks
- Independent outcome verification.
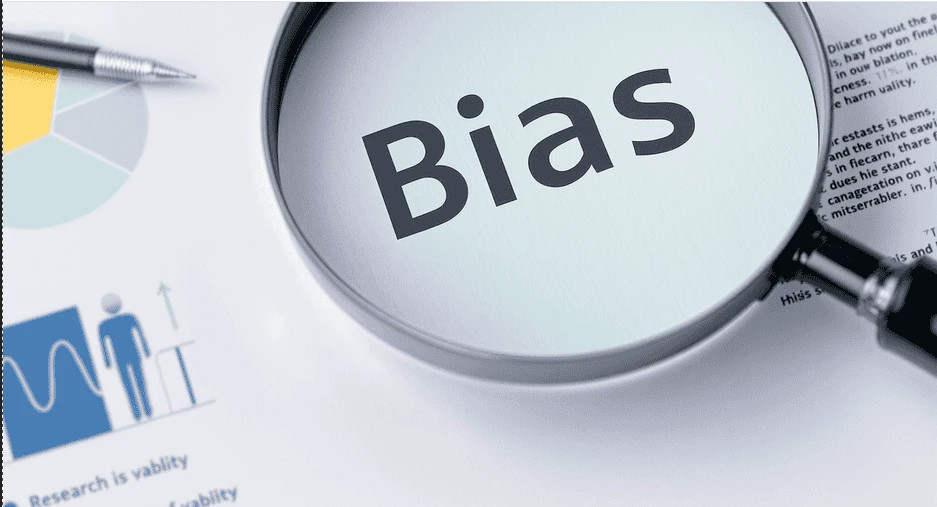
Attrition Bias
Attrition bias occurs when participants drop out of a study before its completion, potentially skewing research results. This type of bias presents significant challenges in longitudinal studies and clinical trials.
Common causes of participant dropout include:
- Loss of interest in the study
- Adverse reactions to treatments
- Relocation to different areas
- Time constraints
- Personal circumstances
- Health complications
The impact of attrition bias becomes particularly severe when:
- Dropout rates differ between study groups
- Participants leave due to treatment-related factors
- Missing data patterns show systematic differences
Prevention Strategies:
- Implement robust follow-up procedures
- Maintain regular contact with participants
- Offer incentives for study completion
- Design realistic study durations
- Create flexible scheduling options
- Document reasons for withdrawal
Missing data analysis techniques help researchers assess the extent of attrition bias. Methods like multiple imputation and sensitivity analyses provide tools to handle incomplete datasets while maintaining statistical validity.
Language Biases
Language bias poses significant challenges in international research studies, especially when working with diverse linguistic populations. This bias manifests in several critical ways:
- Translation Discrepancies: Research instruments translated across languages may lose their original meaning or context, affecting data quality
- Cultural Interpretations: Words and phrases can carry different connotations across cultures, leading to misunderstandings
- Response Patterns: Participants may interpret rating scales differently based on their linguistic background
Research teams conducting multi-site trials face specific language-related challenges:
- Limited access to qualified translators
- Inconsistent terminology across languages
- Varying literacy levels among participants
- Regional dialects and colloquialisisms
To minimize language bias, researchers can implement these strategies:
- Employ certified translators familiar with medical/research terminology
- Use back-translation techniques to verify accuracy
- Conduct pilot studies with multilingual participants
- Create standardized glossaries for key terms
- Include native speakers in the research team
Language bias can significantly impact data quality and research validity. Studies involving multiple languages require careful planning and rigorous translation protocols to maintain consistency across all research sites.
Conclusion
Research bias poses significant challenges to scientific integrity and the validity of study findings. Identifying and addressing these biases is essential for producing reliable, credible research that advances knowledge across disciplines.
Key Strategies to Prevent Research Bias:
- Design robust methodologies with clear protocols and standardized measurement tools
- Implement random sampling and assignment techniques
- Use blinding procedures when appropriate
- Pre-register study protocols
- Maintain detailed documentation of all research procedures
- Engage in peer review throughout the research process
- Consider multiple perspectives and alternative explanations
- Report all findings, regardless of outcome
Best Practices for Bias Reduction:
- Establish clear research questions and objectives before beginning
- Create comprehensive study designs that account for potential confounding variables
- Use validated measurement instruments
- Employ appropriate statistical methods
- Document any limitations or potential sources of bias
- Practice transparency in reporting methods and results
The pursuit of unbiased research requires constant vigilance and commitment to methodological rigor. By understanding and actively addressing different types of bias, researchers can enhance the quality and reliability of their work. This knowledge empowers researchers to design better studies, collect more accurate data, and draw more valid conclusions - ultimately contributing to the advancement of scientific knowledge.
FAQs (Frequently Asked Questions)
1. What is research bias and why is it significant in academic studies?
Research bias refers to systematic errors or deviations from the truth in study design, data collection, analysis, or reporting that can affect the validity and reliability of research findings. Understanding different types of research bias is crucial for ensuring the accuracy and generalizability of academic study outcomes.
2. How does design bias affect research studies and what strategies can minimize it?
Design bias occurs due to organizational issues or flaws in research methods, such as flawed experimental designs or inadequate control groups. It can compromise study validity and generalizability. To minimize design bias, researchers should carefully plan studies with robust designs and appropriate controls during the planning phase.
3. What causes sample bias and how can researchers obtain representative samples?
Sample bias arises when participant selection is non-representative, often due to small sample sizes or biased recruitment methods. This leads to limited external validity and distorted effect sizes. Techniques like stratified random sampling help researchers obtain representative samples and reduce sample bias.
4. What is performance bias and how does the Hawthorne effect influence research outcomes?
Performance bias involves changes in participant behavior due to observation or researcher expectations, including differential treatment between groups. The Hawthorne effect refers to participants altering their behavior because they know they are being observed, which can impact results. Blinding participants and researchers to group allocation helps address performance bias.
5. Can you explain reporting bias and methods to reduce its impact on scientific literature?
Reporting bias occurs when only certain results, often positive outcomes, are selectively reported based on beliefs or expectations. This undermines the credibility of research literature. To reduce reporting bias, researchers can pre-register study protocols and follow comprehensive reporting guidelines like CONSORT.
6. What are confounding biases and how do they affect causal inference in observational studies?
Confounding biases arise when extraneous variables correlate with both the exposure and outcome, potentially leading to incorrect conclusions about causal relationships. They pose significant challenges in observational studies by distorting true associations. Careful study design and statistical control methods are essential to mitigate confounding biases.